In recent years, de novo design of high-affinity protein-binding proteins has become a reality, solely based on target structural information. However, there is still significant room for improvement, as the overall design success rate remains low. In this post, we discuss our lab’s latest open-access research paper published in Nature Communications, which explores the augmentation of energy-based protein binder design using deep learning, resulting in a nearly tenfold increase in design success rates.
This project was led by graduate student Nate Bennett and research scientist Brian Coventry, PhD. It included collaborators from Ghent University in Belgium.
Main Findings
Our experiments demonstrated that by combining physically-based methods with deep learning-based approaches, trained on large numbers of protein structures, we can achieve significant improvements in the one-sided protein-interface design challenge. By using AlphaFold2 or RoseTTAFold to assess the probability that a designed sequence adopts the designed monomer structure and the probability that this structure binds the target as designed, we observe a tenfold increase in design success rates.
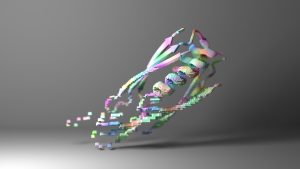
Additionally, we found that using ProteinMPNN for sequence design, instead of Rosetta, significantly increased computational efficiency. Our findings reveal the considerable potential of deep learning in improving protein binder design and the likelihood that the designs will fold and bind as intended.
Challenges and Future Outlook
While our protocol represents an order-of-magnitude improvement over the previous state-of-the-art, there is still much to be understood about interface energetics. Success rates among the targets remain low (<1%), and no binders were identified for certain protein targets. Moreover, there is room for improvement in designing high-affinity binders, as the initially generated binders are in the high nanomolar affinity range.
“Challenges remain, but the rapid pace of advancements in this field promises even more astonishing results in the near future.”
Co-lead author Nate Bennett
Given the rapid progress in the field, we anticipate further increases in design success rates and affinities in the near future, making computational protein design methods even more powerful compared to empirical selection methods for generating affinity reagents and therapeutic candidates. An open question remains whether the future advancements will come from the integration of deep learning and physically-based methods or from deep learning alone. In any case, the field is in for some exciting developments.
Conclusion
Our lab’s latest research demonstrates the considerable potential of deep learning in revolutionizing protein binder design. By combining physically-based methods with deep learning-based approaches, we achieved a tenfold increase in design success rates and improved computational efficiency. While challenges still persist, the rapid progress in the field holds promise for even more powerful computational protein design methods in the near future.
Funding
This work was supported by The Donald and Jo Anne Petersen Endowment for Accelerating Advancements in Alzheimer’s Disease Research, a gift from Microsoft, the Audacious Project at the Institute for Protein Design, a grant from DARPA supporting the Harnessing Enzymatic Activity for Lifesaving Remedies (HEALR) Program, the Flanders Institute for Biotechnology, a Strategic Basic Research grant from Research Foundation Flanders, and the Howard Hughes Medical Institute. We thank AWS and Microsoft for generous gifts of cloud computing credits and Texas Advanced Computing Center (TACC) at the University of Texas at Austin for providing the CPU resources for all Rosetta design calculations.